A multimodal journey
Multimodal learning has seen unprecedented improvements over the last decade. The availability of large scale data combined with modeling and compute improvements have been the perfect environment for machine learning models to excel at understanding modalities beyond text. In this talk, we will do a brief review of the history of multimodal learning with special focus on techniques involving vision and audio – from early self-supervised learning techniques such as MMV to large language models such as Gemini that are able to process text, vision and audio combined.
Dr. Adrià Recasens
Staff Research Scientist at Google DeepMind
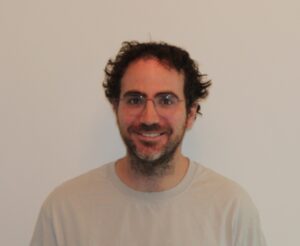