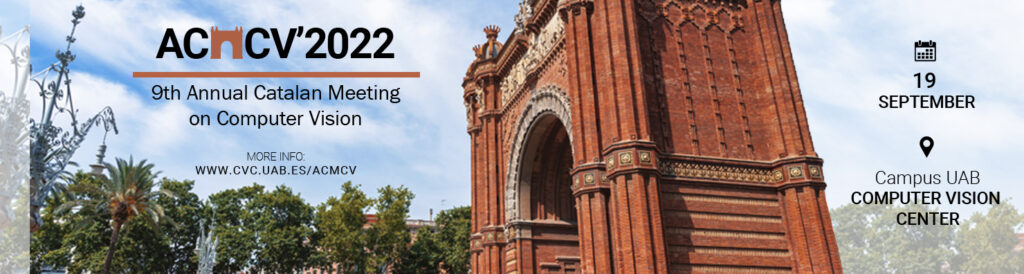
Computer Vision has become a worldwide mainstream research area. Catalonia has an extensive community in the field since most of Catalan universities have research groups dedicated to its study and many small and large companies are leading the development of products using these technologies. The aim of this meeting is threefold:
- To strengthen the Catalan academic and industrial computer vision network.
- To disseminate within the community the most relevant works in that network that have already been published abroad.
- To allow students from the Master in CV (MCV) meet with members of the Catalan CV community and prospective employers.
Dates & Venue:
📑 Submission deadline | September 09, 2022
✍ Registration deadline | September 16, 2022
🗓 ACMCV 2022 | September 19, 2022
📍 Computer Vision Center & UAB School of Engineering
Program:
14:00 – 17:30 h: Master thesis presentations
17:30 – 19:00 h: Reception, Poster session and Coffee
19:00 – 19:45 h: Keynote Talk: Dr Antonio Torralba (Massachusetts Institute of Technology) – “Dataset distillation and Learning to see by looking at noise“
19:45 – 20:30 h: Spotlight session of Best ACMCV 2022 Papers
20:30 – 20:45 h: Awards and Closing
20:45 h: Drinks
Keynote Talk:
Dataset distillation and Learning to see by looking at noise | Dr Antonio Torralba | Delta electronics Professor and head of the AI+D faculty at the Department of Electrical Engineering and Computer Science (EECS) at the Massachusetts Institute of Technology (MIT)
The importance of data in modern computer vision is hard to overstate. The ImageNet dataset, with its millions of labelled images, is widely thought to have spurred the era of deep learning, and since then the scale of vision datasets has been increasing at a rapid pace. These datasets come with costs: curation is expensive, and they inherit human biases. To counter these costs, interest has surged in unsupervised learning as it avoids the curation efforts, or using virtual worlds. In this talk, Dr Antonio Torralba covered two projects that try to reduce the size of datasets needed to train computer vision systems. He first talked about dataset distillation, the task of synthesizing a small dataset such that models trained on it achieve high performance on the original large dataset. A good small distilled dataset is not only useful in dataset understanding but has various applications (e.g., continual learning, privacy, neural architecture search, etc.). Finally, he went a step further and ask if we can do away with real image datasets entirely, instead of learning from noise processes. Findings show that good performance on real images can be achieved even with training images that are far from realistic.
Dataset distillation – https://georgecazenavette.github.io/mtt-distillation/
Learning from noise – https://mbaradad.github.io/learning_with_noise/
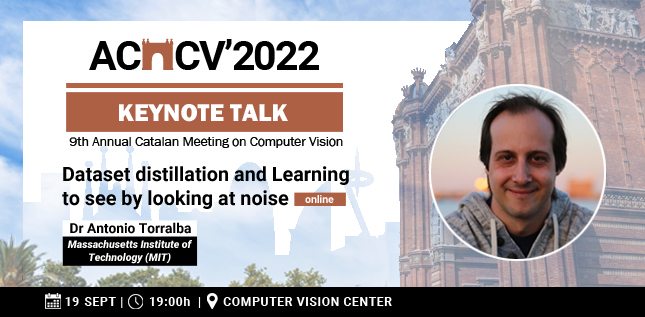
Resources:
- Mastes Thesis Presentations | List of abstracts
- Poster Session | List of papers
- Spotlight Session | List of papers
- ACMCV 2022 Program | Download the program
Organizers:
Organizing Committee:
- Maria Vanrell, CVC & UAB
- Josep Lladós, CVC & UAB
- Núria Martínez, CVC
- Laura Martín, CVC
- Jorge Clusa, CVC
Program Committee:
- Kai Wang, UAB & CVC
- Carles Ventura, UOC
- Alejandro Cartas, UPF