Tuesday, September 17
◼ Track 1: AGRICULTURE | Room Q3/0007
◼ Track 2: DOCUMENT ANALYSIS | Q3/0003
◼ Track 3: EMOTIONS | Q3/0009
◼ Track 4: MEDICAL APPLICATIONS | Q3/0011
◼ Track 5: NEW ARCHITECTURES | Q3/0013
◼ Track 6: AUTONOMOUS DRIVING | Q3/0007
📌
Escola Enginyeria, Edifici Q, UAB
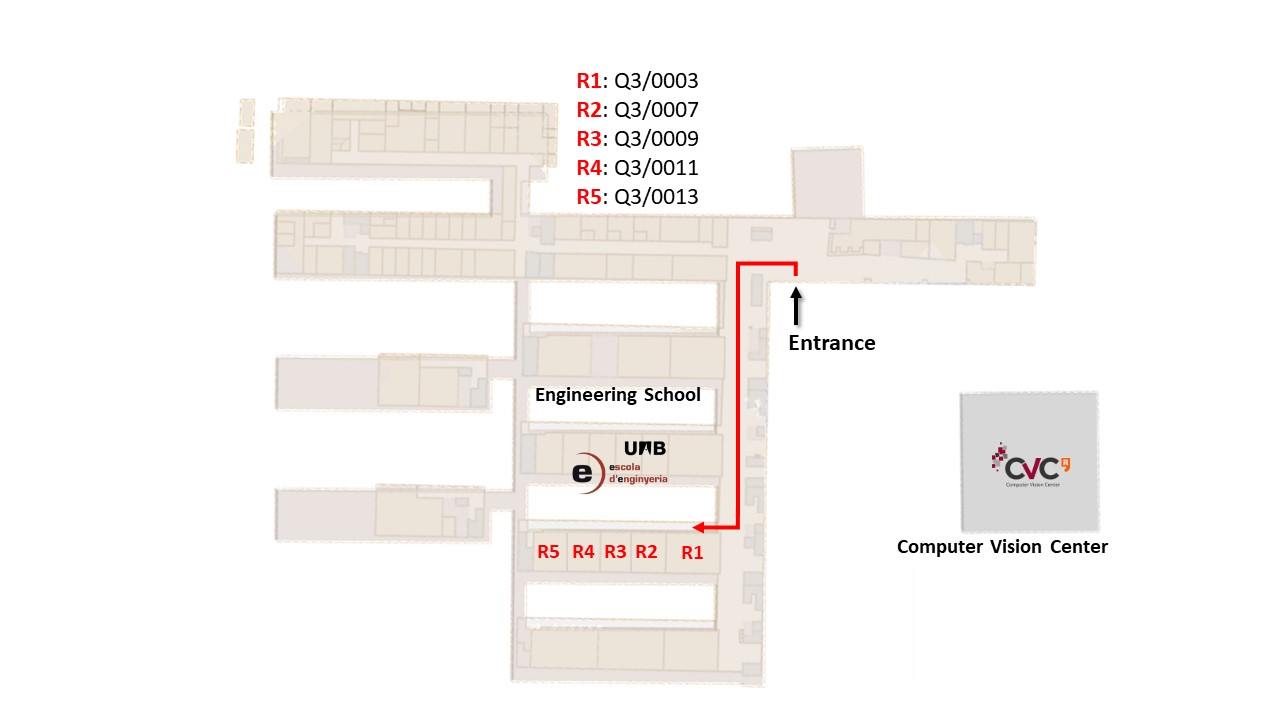
14:00 – 14:45
Advisor/s: Daniel Ponsa (UAB)
Abstract:
This research introduces a novel approach to improving fruit tree management tasks, such as branch pruning and fruit counting, by leveraging 3D radiance fields constructed from video sequences using 3D Gaussian splatting. Traditional video-based methods often struggle with the complexities of tree canopies, leading to inaccuracies. Our approach enhances the accuracy and efficiency of these tasks by developing a pipeline that transforms 2D videos into 3D radiance fields, incorporating techniques like feature distillation and object segmentation for precise 3D analysis. Additionally, we propose a feature field distillation method, Feature 3DGS, and Gaussian Grouping to enable detailed object identification within the 3D space. We also explore integrating language models for intuitive scene interaction, demonstrating that radiance fields offer a robust and versatile solution for advanced fruit tree management
Committee:
President: Josep R. Casas (UPC)
Secretary: Ramon Baldrich Caselles (UAB)
Vocal: Javier Ruiz Hidalgo (UPC)
Advisor/s: Oriol Ramos (UAB)
Abstract:
In Historical Demography, the reconstruction of individual life courses involves linking data of the same person that appear in different documents, such as baptism, and marriage certificate, among others. However, poor data quality can make record linkage challenging. The conservation status of original documents, the scanning process, and the large number of similar demographic values in names, ages, or addresses are just some of the factors that can affect data quality. This project proposes the construction of a visual graph that contains the information of each individual. In this graph, there are two types of nodes: one contains demographic representations and the other contains the representation of the individual constructed from the nodes with demographic information. This graph allows us to learn about a representation space where individuals who have similar demographic information are nearby. To build the graph, a dataset has been generated from the information of each individual that appears in the collections of the different censuses of San Feliu del Llobregat in the XIX-XX centuries. The consistency of the graph is subsequently evaluated by link discovery between individuals that appear in the population of Sant Feliu at different times. The first experiments show satisfactory results in the construction of the graph and the learning of the representation space, although there is still room for improvement. Visual inspection of the results shows that the learned metric space is consistent with the representation sought. Preliminary experiments in the link discovery task reinforce the starting hypothesis
Committee:
President: Ernest Valveny Llobet (UAB)
Secretary: Josep Llados Canet (UAB)
Vocal: Mohamed Ali Souibgui (CVC-UAB)
Advisor/s: Jordi Sanchez Riera (IRI)
Abstract:
This thesis introduces a novel approach for enhancing the expressiveness of speech-driven facial animation by incorporating
emotion-aware head rotations. Building upon recent advancements in generating realistic lip movements and facial expressions
from speech, we address the often-overlooked aspect of head motion in realistic animations, which plays a crucial role in conveying
emotions during natural communication. Our method leverages a dedicated Transformer decoder to predict sequences of head
rotations directly from the emotional content embedded in the speech signal. This is achieved by first disentangling emotional
features from linguistic information using pre-trained audio encoders. This approach acknowledges that head pose is not just
incidental but a deliberate reflection of the speaker’s emotional state. This emotion-guided approach, implemented within the
Rotating Multi-modular Mesh Animator (RoMMA) framework, contributes to a significant advancement in the expressiveness
of virtual avatars
Committee:
President: Alicia Fornes (UAB)
Secretary: Ismael Benito-Altamirano (UOC)
Vocal: Cristina Bustos (Universitat Oberta de Catalunya)
Advisor/s: Oscar Camara (UPF)
Abstract:
This thesis presents the implementation and evaluation of an active learning pipeline aimed at enhancing the segmentation cardiac structures from computerised tomography (CT) scans, focusing on the left atrium (LA), right atria (RA), circumflex branch of the left coronary artery (Cx), and fossa ovalis (FO), all relevant to left atrial appendage occlusion (LAAO) procedures. The system integrates a nnU-Net segmentation model into the VIDAA platform, a web-based tool for pre-planning LAAO procedures, using active learning to improve segmentation accuracy through expert-reviewed corrections iteratively. MedSAM, a recent foundational model for segmenting medical images, was also tested to streamline the ground truth generation process, reducing the effort for manual segmentations. Integrating the VIDAA platform required work at different levels, from the back-end and infrastructure level, using Kubernetes for containerised deployment, to front-end and web development for interactive viewers, as well as database management. By optimising segmentation accuracy and workflow efficiency, this system aims to enhance preoperative planning for LAAO procedures, ultimately improving decision-making and increasing the admissible throughput of patients.
Committee:
President: Simone Tassani (UPF)
Secretary: Jordi Sanchez Riera (IRI)
Vocal: Montse Pardàs (UPC)
Advisor/s: Fernando Vilariño (UAB), Matias Bilkis (CVC/UAB)
Abstract:
In recent years, efforts to develop fault-tolerant quantum hardware have been intensifying driven by the theoretical potential of quantum computing. On the other hand, the advancement in classical computational power, specifically the development of more performant graphics processing units, facilitated progress in the field of machine learning with generative models gaining extreme popularity and diffusion models particularly emerging as one of the favorites for the task of image generation. In this context, we formulated the question of what would be a pertinent quantum machine learning approach to the diffusion models that would make use of the quantum properties. This thesis explores two architectures for the denoising step of the diffusion models, a fully quantum one and a hybrid classical-quantum one. We do so by analyzing their generative properties from the perspective of the generative learning trilemma, drawing comparisons to their classical counterpart, and explaining their possible advantages and limitations
Committee:
President: Xavier Otazu (UAB)
Secretary: Raül Pérez (UPC)
Vocal: Sanket Biswas (CVC-UAB)
14:45 – 15:30 h
Advisor/s: Jordi Gené Mola (UdL), Javier Ruiz Hidalgo (UPC)
Abstract:
Accurate detection of apples in orchards is crucial for efficient harvest planning and crop management. However, the non-invasive detection of apples using 3D point clouds present challenges, particularly due to the complexity of the orchard environment, including occlusions and varying sensor modalities. This work introduces a novel approach for apple detection in 3D point clouds using a Deep Neural Network (DNN) architecture based on transformers, specifically the 3DETR model. The proposed framework is capable of detecting apples and providing precise 3D bounding boxes around each detected fruit. The network was trained and evaluated on two distinct datasets, utilizing both RGB and LiDAR modalities. The results demonstrate the electiveness of the approach, achieving a mean Average Precision (mAP) of 75.86% at IoU 50 on the RGB dataset and a mAP of 82.4% at IoU 50 on the reflectance dataset. These results underscore the potential of transformer-based models like 3DETR in enhancing the accuracy and reliability of fruit detection tasks in complex agricultural environments
Committee:
President: Josep R. Casas (UPC)
Secretary: Jose Luis Gomez Zurita (CVC-UAB)
Vocal: Daniel Ponsa (UAB)
Advisor/s: Alicia Fornes (UAB), Xavier Otazu (UAB)
Abstract: Spiking Neural Netowrks (SNN) are an energetically efficient and biologically plausible paradigm for the creation of artificial neural networks, in which information transmitted between layers is composed exclusively of discrete spikes. In this project, we will explore the applications of SNNs to the recognition of individual handwritten characters with a focus on maintaining the the biological plausibility of both the network architecture as well as the learning algorithm. We implement a two layer network capable of classifying images of digits encoded as poisson spike-trains, trained exclusively using a spike-time-dependent-plasticity (STDP) rule as the learning method, making it completely unsupervised. The network uses a leaky-integrate-and-fire (LIF) neuron model with conductance-based synapses, and evolution of the variables defined in the models is obtained through integration over discrete time-steps, similarly to a clock-driven simulation
Committee:
President: Oriol Ramos (UAB)
Secretary: Ramon Morros (UPC)
Vocal: Marta Saiz Vivó (UPF)
Advisor/s: Jordi Sanchez Riera (IRI)
Abstract:
League of Legends is one of the most popular esports games, with its highly competitive gameplay demanding both strategic precision and real-time decision-making. Analyzing gameplay events—such as team fights and objective captures—is crucial for optimizing strategies and improving team performance. However, the influence of emotions on player performance cannot be understated. Understanding player emotions during critical moments offers valuable insights into their psychological state, which can significantly affect in-game decisions and outcomes.
This study focuses on developing advanced techniques for real-time highlight detection and information extraction tailored for esports, particularly in League of Legends. By integrating visual cues from optical flow and color intensity with audio signal processing through Convolutional Neural Networks (CNNs), we detect key gameplay highlights. We then analyze this data and correlate it with player emotions using facial recognition. Our findings suggest that this approach offers valuable insights for coaching and strategy optimization. However, challenges such as limited facial visibility due to gaming headsets or neutral expressions can affect the accuracy of emotion detection. Future work could enhance this by incorporating player voice communications (voice comms), providing a richer dataset for more precise emotion analysis and a deeper understanding of how emotions influence gameplay
Committee:
President: Simone Tassani (UPF)
Secretary: Ismael Benito-Altamirano (UOC)
Vocal: Guillem Martínez Sánchez (UB)
Advisor/s: Montse Pardàs (UPC), Veronica Vilaplana (UPC)
Abstract:
Prostate cancer (PCa) is the second most common cancer in men worldwide, with early detection of clinically significant cases (csPCa) being crucial for improving patient outcomes. While traditional diagnostic methods have limitations, biparametric MRI (bpMRI) has emerged as a preferred approach for detecting high-risk csPCa. However, the interpretation of bpMRI can be subjective and inconsistent among radiologists, which may be mitigated with the introduction of computer-aided diagnosis (CAD) systems. This study explores segmentation and classification algorithms and demonstrates how deep learning models trained on large, diverse datasets are able to generalize better. In this context, we present a deep learning-based CAD system that uses a nnU-Net model for prostate segmentation and incorporates an integrated classification head for lesion detection. On the ProstateX testing data, the proposed model achieves a Dice Similarity Coefficient (DSC) of 0.93 and 0.84 for the Central Zone+Transition Zone and Peripheral Zone of the prostate, respectively, a DSC of 0.67 for csPCa lesion segmentation, and an Area Under the Receiver Operating Characteristic Curve (AUROC) of 0.86 for the classification task
Committee:
President: Jordi Sanchez Riera (IRI)
Secretary: Lei Kang (CVC-UAB)
Vocal: Carles Ventura Royo (UOC)
Advisor/s: David Serrano Lozano (UAB/CVC), Javier Vazquez Corral (UAB)
Abstract:
Color naming, the process of categorizing colors into universal terms, plays a significant role in how humans perceive and describe images. Leveraging this concept, this thesis integrates color naming probability maps into transformer-based models to enhance image retouching. By embedding these maps into the deep learning pipeline of models like Restormer and PromptIR, the study aims to replicate the nuanced adjustments made by expert retouchers. The results demonstrate that incorporating color naming improves color accuracy and visual quality, providing a novel approach to automated image enhancement
Committee:
President: Raül Pérez (UPC)
Secretary: Jordi Gonzàlez (CVC-UAB)
Vocal: Maria Vanrell Martorell (UAB)
15:30 – 16:15
Track 1: 3D VISION | Advisor/s: Bogdan Raducanu (UAB) | Abstract: Accurately understanding and interpreting 3D scenes in robotic environments is a critical task for various applications, such as object manipulation and scene navigation. This paper presents an improved methodology for 3D scene understanding using as reference the CLIP-Fields framework, which integrates recent advancements in vision and language models for robotic applications. Our key contributions include: the adoption of a larger closed-set vocabulary, efficient memory usage changes in the training pipeline, analysis of various pre-trained models with altering inputs, improved query retrieval in the inference pipeline, and the creation of a dynamic point cloud to handle scene changes.
Advisor/s: Ernest Valveny Llobet (UAB)
Abstract:
This thesis explores the application of advanced vision-language models, specifically UniChart, MatCha, and Florence-2, within a Visual Question Answering (VQA) framework for chart analysis. The primary objective was to evaluate the effectiveness of these models in performing tasks related to chart classification and VQA, particularly in handling the complex integration of visual and textual data. Two main experiments were conducted: the first focused on chart classification using both the DocFigure and YouTubeCharts datasets, while the second extended the scope to chart-based VQA using the YouTubeCharts dataset. The results demonstrate that while all three models can achieve high accuracy in straightforward classification tasks, their performance varies significantly when tasked with answering diverse questions about charts. Florence-2 consistently outperformed the other models, especially in tasks requiring more complex reasoning and context-specific interpretation. The findings highlight the potential of vision-language models for automated chart analysis, suggesting that while a unified VQA framework is feasible, task-specific fine-tuning can lead to better performance.
Committee:
President: Ramon Morros (UPC)
Secretary: Oriol Ramos (UAB)
Vocal: David Serrano Lozano (UAB/CVC)
TRACK 3 - Q3/0009 Diana Tat | A study of automatic emotion perception in children aged 3 to 5 years.
Advisor/s: Àgata Lapedriza Garcia (UOC), Lucrezia Crescenzi-Lanna (UOC)
Abstract:
Emotion perception is an area of computer vision that has been explored mainly with adults, while underrepresented populations (infants and elderly) have been left out of the studies. This thesis brings the children to the spotlight, more specifically those aged between 3 and 5 years old. While we can find a lot of open-source datasets with adults, there are very few datasets that include teenagers or children, especially so young. Studying emotion perception on such a young population opens some interesting applications, such as educational implementations, pediatric monitoring and health practices, understanding the childhood development stages, strengthening parent-children interaction, to name a few. During this study, we test different approaches of emotion perception on children. The first one is testing a standard method that has been used in previous studies for emotion classification, which is the OpenFace tool. It is an open-source software that detects facial landmarks and head pose estimation directly on the input video, and predicts Action Units (AUs). While this software is very handy to use, it doesn’t provide a direct method to obtain facial expression or emotion labels. Because of that, we will also use state-of-the-art facial emotion prediction models that are prior trained on adults and evaluate their performance on kids. Additionally, we retrain and fine-tune these models on children data. To test the different methods we use two datasets. The first one is EmoReact, which is an open dataset that contains children from 4 to 14 years old and that was created by cutting some videos where kids reacted to certain things and posted to the React2 channel in Youtube. The second one is called App2Five and it is a custom dataset, containing recordings captured in a school with children playing with educational app. Unlike EmoReact, which was labeled by external experts, for this dataset we created the labels after some sessions of human training where we established a procedure of how to watch the videos, with and without sound, watch them in slow-motion and watch the same video with context added. Overall, the goal of the thesis is to evaluate the following hypothesis: (1) whether OpenFace, which is trained on adults, can correctly detect children's emotions and to see if the different techniques are better or not; (2) whether state-of-the-art models trained on adults can effectively perceive emotions in young children; and (3) whether fine-tuning or retraining these models can lead to improved performance on this specific task
Committee:
President: Javier Vazquez Corral (UAB)
Secretary: Julio Cezar Silveira Jacques Junior (UB)
Vocal: Álvaro José Bocanegra Pérez (UPF)
Advisor/s: Simone Tassani (UPF)
Abstract:
The number of people in need of daily rehabilitation exercises continues to rise steadily, due to the aging of the population
and the use of post-stroke therapies. The creation of rehabilitation exercises controlled by pose estimation models provides a new
tool to support specialists in reaching a larger number of patients at the same time. Through this work we have created a series of
hand and body rehabilitation exercises based on MediaPipe pose estimation models. In addition, these exercises have been tested
by a sample of subjects concluding with a correct performance of all exercises
Committee:
President: Joan M Núñez do Rio (Universitat Oberta de Catalunya)
Secretary: Jordi Sanchez Riera (IRI)
Vocal: Simone Balocco (UB)
Advisor/s: Jordi Gonzàlez (CVC-UAB), Pau Rodriguez (UAB)
Abstract:
This MSc thesis investigates the improvement of reliability and fairness in text-to-image diffusion models, particularly addressing the issues of concept spillage and societal biases introduced by text encoders like CLIP. We explore for the first time the use of self-conditioning approaches for diffusion models that adjusts neuron activations in the text encoder, allowing for effective mitigation of these problems without necessitating a full model retrain. Our method involves targeted interventions at the inference stage, enhancing both the precision and ethical aspects of image generation. Extensive experiments conducted across multiple versions of the Stable Diffusion model demonstrate that these interventions significantly diminish the occurrence of unintended concept representations and biases. The results indicate not only improved performance but also more fairness in the generated images. This strategy presents a computationally efficient pathway to enhance generative model reliability, setting a groundwork for future developments in ethical AI practices. This research contributes to the broader conversation about the responsibilities and capabilities of AI in adhering to ethical standards, thus pushing the boundaries of what is possible in the domain of generative models
Committee:
President: Javier Ruiz Hidalgo (UPC)
Secretary: Lluís Gómez Bigorda (UAB)
Vocal: Cristina Bustos (Universitat Oberta de Catalunya)
Advisor/s: Antonio López Peña (UAB), Gabriel Villalonga (CVC/UAB)
Abstract:
This paper introduces CILTM (Conditional Imitation Learning with Token Memory), a significant advancement in the Conditional Imitation Learning (CIL) architecture for autonomous driving models, achieved by embedding memory mechanisms through the Token Turing Machine (TTM) framework. By incorporating memory cells, CILTM gains the ability to retain and leverage contextual cues, addressing key challenges in conventional end-to-end driving models. Given the computational demands, we focus on critical classification tasks such as lane positioning, speed modulation, and steering direction. Through rigorous experimentation, including hyperparameter optimization, CILTM demonstrates a clear performance edge over the baseline CIL++ architecture. These results illuminate the path forward for more adaptive and context-aware driving systems, positioning memory-augmented architectures like CILTM as a foundation for the next generation of autonomous vehicles
Committee:
President: Josep R. Casas (UPC)
Secretary: Fernando Vilariño (UAB)
Vocal: Jose M Porta (Institut de Robòtica i Informàtica Industrial)
16:15 – 17:00
Advisor/s: Dimosthenis Karatzas (UAB), Lei Kang (CVC-UAB)
Abstract:
Multi-Page Document Visual Question Answering presents a significant challenge compared to Single-Page Document Visual Question Answering due to the increased complexity of extracting relevant information across multiple pages. State-of-the-art methods typically process these documents at the page level, sequentially aggregating information in either a hierarchical or recurrent manner. This process is computationally intensive and often impractical for longer documents and standard GPU hardware. To overcome these limitations, we propose a novel approach that treats the entire multi-page document as separate channels, enabling more efficient and scalable processing. Extensive experiments demonstrate the effectiveness of our method, showing that it can be successfully applied with acceptable performance in real-world scenarios
Committee:
President: Ernest Valveny Llobet (UAB)
Secretary: Coloma Ballester (UPF)
Vocal: Sanket Biswas (CVC-UAB)
Advisor/s: Àgata Lapedriza Garcia (UOC)
Abstract:
This paper introduces TinyEmo, a family of small multi-modal language models for emotional reasoning and classification. Our approach features: (1) a synthetic emotional instruct dataset for both pre-training and fine-tuning stages, (2) a Metric Projector that delegates classification from the language model allowing for more efficient training and inference, and (3) a semi-automated framework for bias detection. TinyEmo is able to perform emotion classification and emotional reasoning, all while using substantially fewer parameters than comparable models. This efficiency allows us to freely incorporate more diverse emotional datasets, enabling strong performance on classification tasks, with our smallest model (700M parameters) outperforming larger state-of-the-art models based on general-purpose MM-LLMs with over 7B parameters. The Metric Projector allows for interpretability and indirect bias detection in large models without additional training, offering an approach to understand and improve AI systems
Committee:
President: Ismael Benito-Altamirano (UOC)
Secretary: Jordi Sanchez Riera (IRI)
Vocal: Lei Kang (CVC-UAB)
Advisor/s: Montse Pardàs (UPC), Veronica Vilaplana (UPC)
Abstract:
This study explores the synthesis of prostate MRI images using Generative Adversarial Networks, focusing on the StyleGAN2-ADA architecture. While significant progress has been made in medical image generation, understanding and controlling the latent space within these models remains challenging. This research addresses this gap by analysing various model configurations, including conditional and masked models, trained on different MRI slice ranges. Principal Component Analysis (PCA) is applied to investigate the latent space, revealing how different factors influence image quality and lesion representation.
The findings show that simpler models generally achieve superior image quality. Moreover, while masking can enhance lesion visibility, it can sometimes reduce realism. Analysis of the latent space revealed distinct movements, including anatomical shifts and lesion growth, which are often entangled. Additionally, controlled sampling along latent directions associated with lesion growth demonstrates significant potential for generating images with varying degrees of lesion presence.
Committee:
President: Joan M Núñez do Rio (Universitat Oberta de Catalunya)
Secretary: Simone Balocco (UB)
Vocal: Oscar Pina (Universitat Politècnica de Catalunya)
Advisor/s: Antonio Agudo (UPF), Marc Gutiérrez Pérez (UPC)
Abstract:
Predicting fouls in soccer remains challenging despite recent advances in computer vision techniques for player tracking and pose estimation. The small size of the player images and the need to analyze subtle interactions complicate the task. This study presents an approach that aggregates multiple views of actions, focusing on detected players. By integrating video data and bounding box positions from the SoccerNet-MVFoul dataset, our model utilizes a combination of multilayer perceptrons (MLPs) and decoder networks to enhance detection accuracy
Committee:
President: Jordi Gonzàlez (CVC-UAB)
Secretary: Ramon Morros (UPC)
Vocal: Artur Xarles Esparraguera (UB)
Advisor/s: Josep R. Casas (UPC), Santiago Royo (UPC)
Abstract:
Robust detection, localization and tracking of objects is essential for autonomous driving. Computer vision has largely driven development based on camera sensors in recent years, but 3D localization from images is still challenging. LiDAR point clouds provide accurate localization in 3D by measuring distance and even 3D object’s shapes, but LiDAR sensors are expensive, data is less semantic, rather sparse and its range is typically limited to 150m. 4D Imaging radars achieve larger ranges up to 300m adding radial velocity (the 4thD) to the objects detected thanks to Doppler effect. However, the returns are even sparser than LiDAR, and less precise in terms of localization, both for range and beam direction (azimuth and elevation). Cost and limited resolution of range sensors still make them a promising addition to video processing. The evolution towards fusion strategies that take into account both the 3D localization capabilities of range sensors and the higher spatial resolution of image data looks certain to happen.
Committee:
President: Javier Ruiz Hidalgo (UPC)
Secretary: Jose M Porta (Institut de Robòtica i Informàtica Industrial)
Vocal: Javier Vazquez Corral (UAB)
17:00 – 17:45
Advisor/s: Simone Balocco (UB)
Abstract:
Cardiovascular diseases continue to be the leading cause of mortality in Europe, with a significant increase in related deaths observed in recent years. Intravascular ultrasound (IVUS) imaging is a commonly used tool for diagnosing and monitoring vascular conditions, and guiding subsequent treatment. The accurate segmentation of structures such as the Lumen and Media in IVUS images is crucial for effective diagnosing of patients. In recent years significant efforts have been made to automate this process using deep neural networks, with existing literature primarily focusing on creation of specialized model architectures, training regimens, and domain-specific augmentations. This study aims to fill a gap in the literature by evaluating the potential of unsupervised pretraining methods to further enhance the performance of IVUS segmentation models using un-annotated data. In recent years scribble annotations have emerged as a popular format of weak supervision for medical image segmentation models. We evaluate the performance of scribble-supervised segmentation methods using a synthetically generated dataset of scribbles and quantify the effect of noise in scribble annotations on final model performance
Committee:
President: Montse Pardàs (UPC)
Secretary: Oscar Pina (Universitat Politècnica de Catalunya)
Vocal: Javier Vazquez Corral (UAB)
Advisor/s: Dimosthenis Karatzas (UAB), Lei Kang (CVC-UAB)
Abstract:
A common trend in many machine learning fields involves scaling up existing architectures to achieve new state-of-the-art results. This approach presents various challenges, from computational limitations that restrict research accessibility, to environmental concerns due to increased energy consumption. In the field of Computer Vision, these issues are particularly pressing given the need to process larger images while preserving their original resolution for scale-sensitive tasks such as reading. In this sense, despite Transformers being extremely powerful, they are inefficient in nature, requiring processing the whole input to produce attention scores. This inefficiency raises the question of whether more efficient attention mechanisms can be developed.
In this work, we introduce an end-to-end visual attention model for multiple object classification. Drawing inspiration from previous RNN-based visual attention models, we propose an updated architecture centered around Transformers. We evaluate the strengths and limitations of this approach by testing its localization and recognition capabilities on increasingly complex multiple digit classification tasks using the SVHN dataset. Our findings suggest potential upgrades and future work hypotheses to enhance the model's scalability and use in more complex tasks
Committee:
President: Fernando Vilariño (UAB)
Secretary: Gabriel Villalonga (CVC/UAB)
Vocal: David Anglada-Rotger (UPC)